A Library for Robot Learning with Physics-based Optimization
We are excited to share our new open-source library PyPose. It is a PyTorch-based robotics-oriented library that provides a set of tools and algorithms for connecting deep learning with physics-based optimization.
PyPose is developed with the aim of making it easier for researchers and developers to build and deploy robotics applications. With PyPose, you can easily create and test various control, planning, SLAM, or any other optimization-based algorithms and connect them with deep learning-based perception algorithm.
Some of the features of PyPose include:
- Differentiable Lie group and Lie algebra such as SO3, SE3, so3, and se3.
- 2nd-order optimizers such as GaussNewton and LevenbergMarquardt.
- Many other useful differential modules including various differentiable filters, such as EKF, UKF, IMU pre-integration, dynamics model, and linear quadratic regulator, etc.
It provides a simple, user-friendly interface that makes it easy to get started with robot learning. Our hope is that PyPose will help researchers and developers to accelerate the pace of innovation in robotics and enable the development of more advanced and capable robots. We look forward to seeing the innovative robotics applications that will be developed using our library.
How to get started?
- To get started with PyPose, simply install it via
pip install pypose
and visit our Tutorial, Documentation, and GitHub repository. We welcome feedback from the community and are open to contributions from developers who want to improve and extend the library.
Who is developing PyPose?
- We currently have more than 50 volunteer developers from more than 16 institutes and 3 continents. The background of the developers are diverse, covering research directions including SLAM, control, planning, and perception.
Why do we call ourselves “PyPose”?
- PyPose targets for robotics, which mainly consists of three tasks: control, planning, and SLAM. Basically, control is to stabilize robot pose/states, planning is to generate future robot pose, and SLAM is to estimate current/past robot pose. Although some state representations are beyond pose, they mostly can be represented with PyTorch’s Tensor. To highlight this uniqueness in robotics, we call ourselves “PyPose”.
Do you have a paper describing this library?
- Yes! Our paper was accepted to CVPR 2023 with title
PyPose: A Library for Robot Learning with Physics-based Optimization.
You may download it from here.
What will we support in the future?
- As promised in the paper, we will support sparse block tensors, sparse Jacobian computation, constrained optimization, etc.
- All the algorithm infrastructures for robotics, especially those needed by SLAM, control, and planning.
How do we get the latest updates regarding future releases?
- Star our GitHub repository.
- Follow our Twitter and LinkedIn.
How to become a PyPose developer?
- Send an email to us at admin@pypose.org and we will invite you to join our Slack, GitHub org, etc. We currently have monthly plenary developer meetings.
- Alternatively, you may also directly open a Github Issue or Discussion.
Who is the main support of the PyPose library?
- We mainly come from academic institutions.
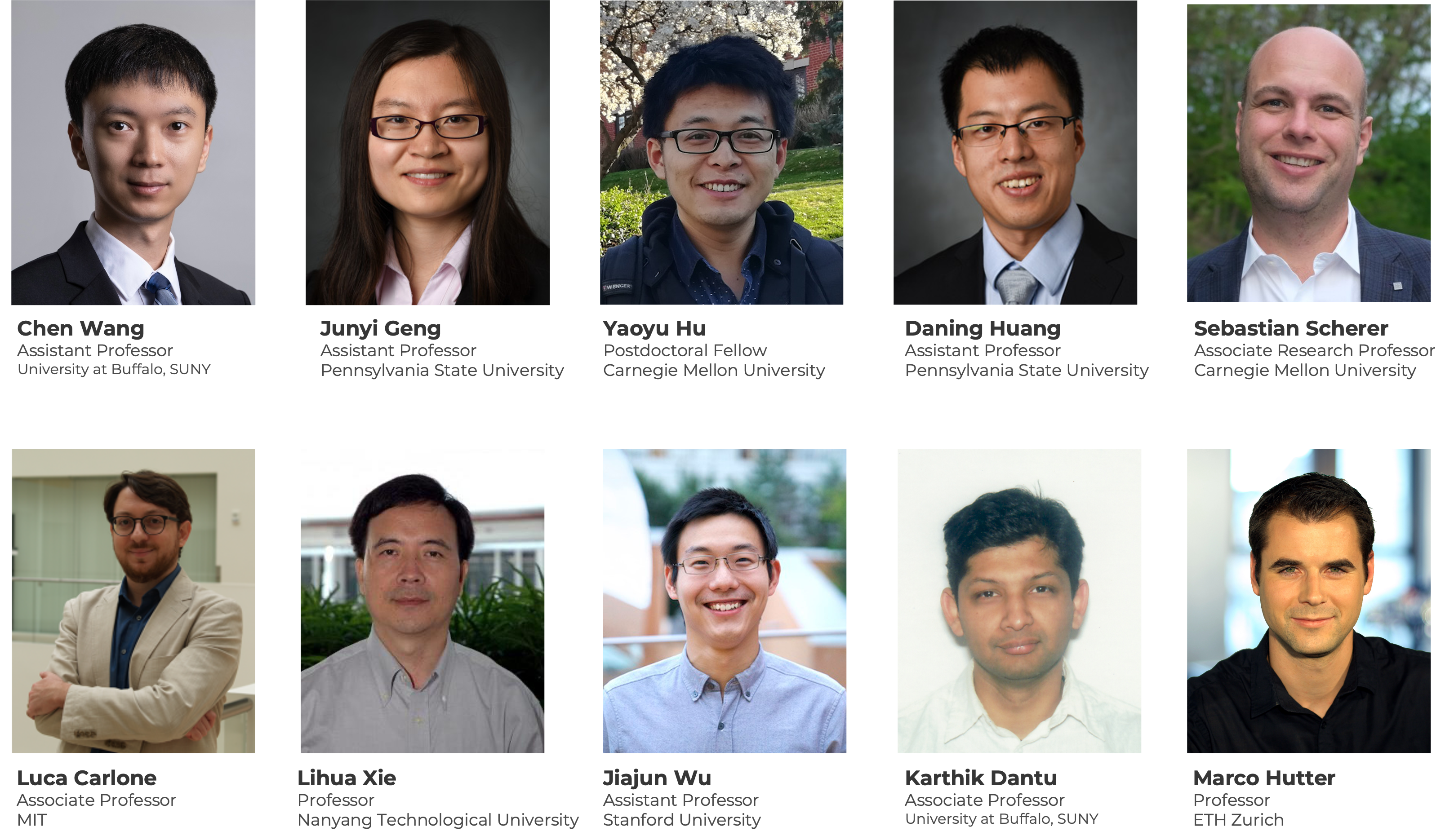
Examples
To boost future research, we provide concrete examples across several fields of robotics, including SLAM, inertial navigation, planning, and control.
SLAM
To showcase PyPose’s ability to bridge learning and optimization tasks, we develop a method for learning the SLAM front-end in a self-supervised manner. As shown in Fig. 1 (a), matching accuracy (reproj. error \(\leq\) 1 pixel) increased by up to 77.5% on unseen sequences after self-supervised fine-tuning. We also show the resulting trajectory and point cloud on a test sequence in below (right). While the pretrained model quickly loses track, the fine tuned model runs to completion with an ATE of 0.63 m. This verifies the feasibility of PyPose for optimization in the SLAM backend.
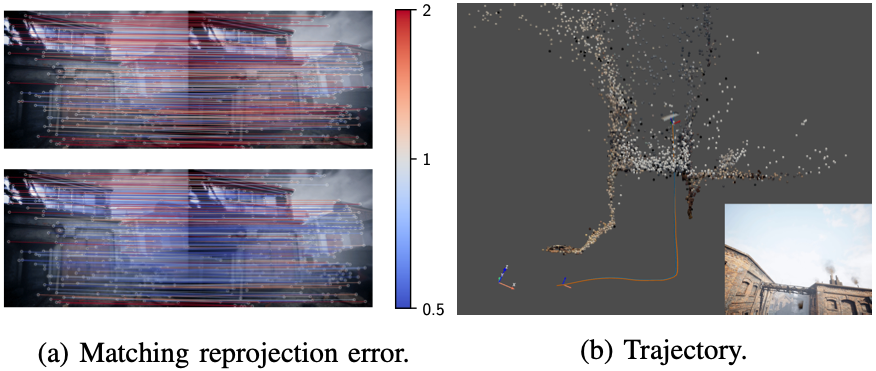
Planning
The PyPose library can be used to develop a novel end-to-end planning policy that maps the depth sensor inputs directly into kinodynamically feasible trajectories. As shown in Fig. 2 (a), our method achieves around \(3\times\) speedup on average compared to a traditional planning framework, which utilizes a combined pipeline of geometry-based terrain analysis and motion-primitives-based planning~Fig. 2 (b). The efficiency of this method benefits from both the end-to-end planning pipeline and the efficiency of the PyPose library for training and deployment. Furthermore, this end-to-end policy has been integrated and tested on a real legged robot system, ANYmal. A planning instance during the field test is shown in Fig. 2 (c) using the current depth observation, shown in Fig. 2 (d).
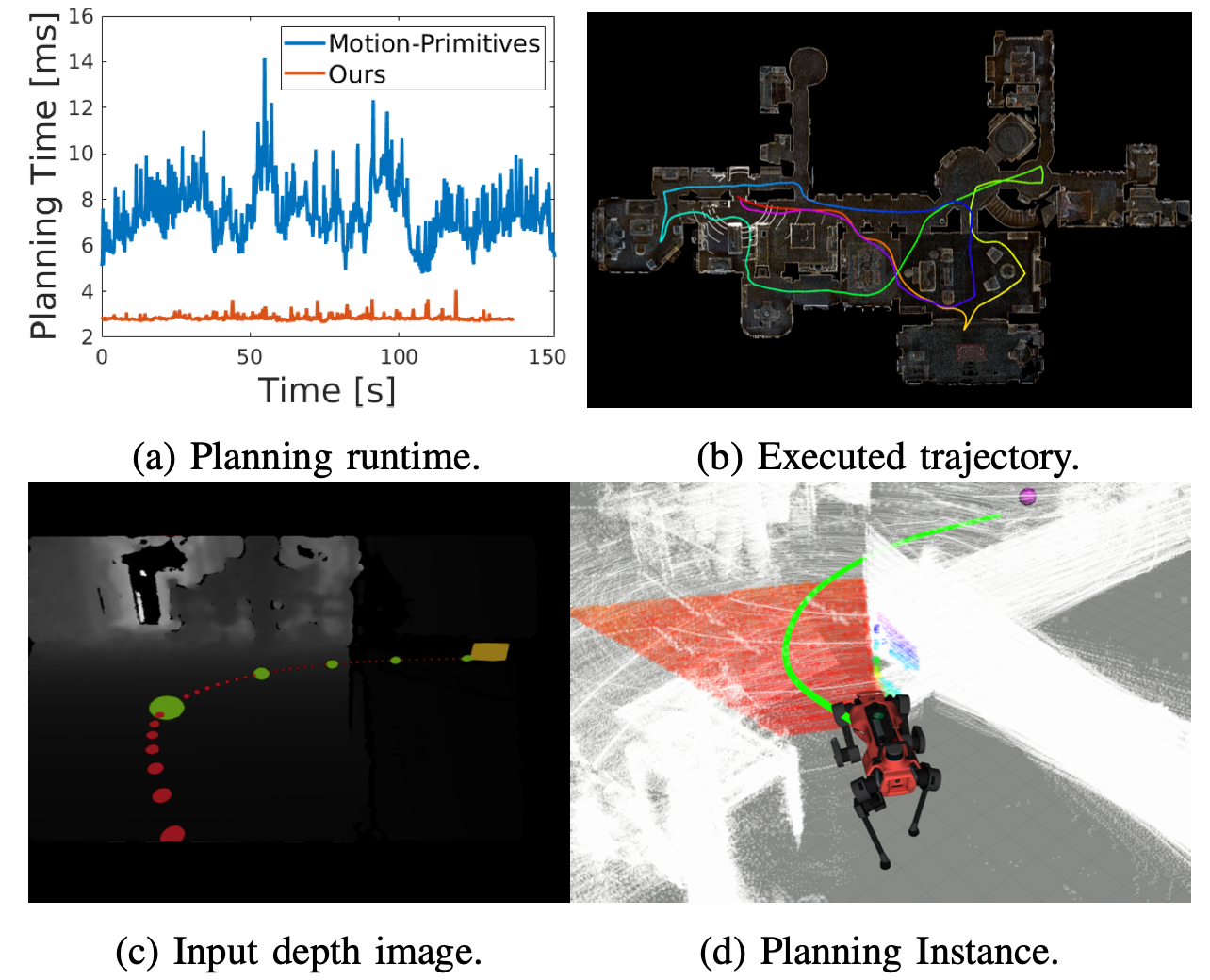
Control
PyPose also integrates the dynamics and control tasks into the end-to-end learning framework. We demonstrate this capability using a learning-based MPC for an imitation learning problem, Diff-MPC, where both the expert and learner employ a linear-quadratic regulator (LQR) and the learner tries to recover the dynamics using only expert controls. We treat MPC as a generic policy class with parameterized cost functions and dynamics, which can be learned by automatic differentiating (AD) through the LQR optimization. Our backward time is always faster because the method Diff-MPC needs to solve an additional LQR iteration in the backward pass. The computational advantage of our method may be more prominent as more complex optimization problems are involved in the learning.
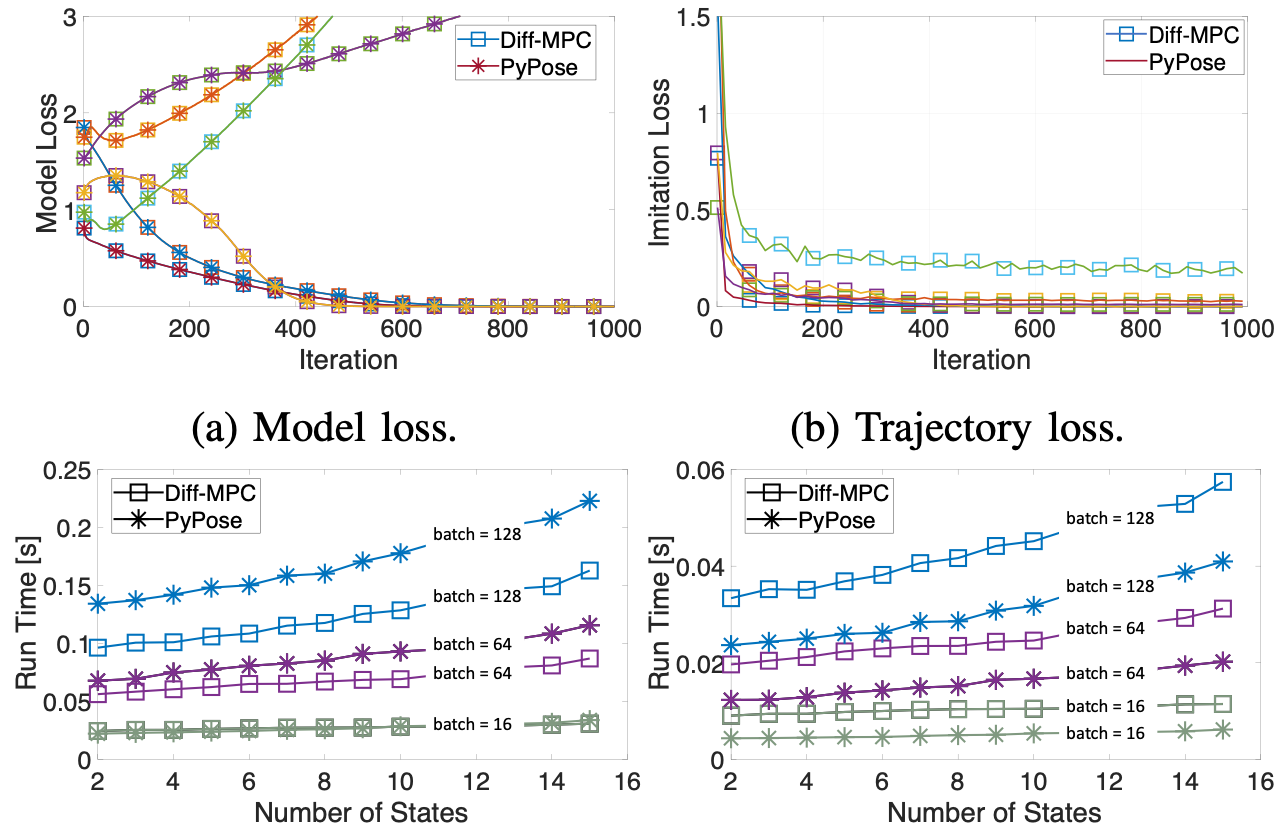
IMU preintegration
To boost future research in this field, PyPose develops an IMUPreintegrator
module for differentiable IMU preintegration with covariance propagation.
It supports batched operation, cumulative product, and integration on the manifold space.
As the example shown in Fig. 4, we train an IMU calibration network that denoises the IMU signals after which we integrate the denoised signal and calculate IMU’s state expressed with LieTensor
including position, orientation, and velocity.
In order to learn the parameters in a deep neural network (DNN), we supervise the integrated pose and back-propagate the gradient through the integrator module.
Our method has a significant improvement on both orientation and translation, compared to the traditional method.
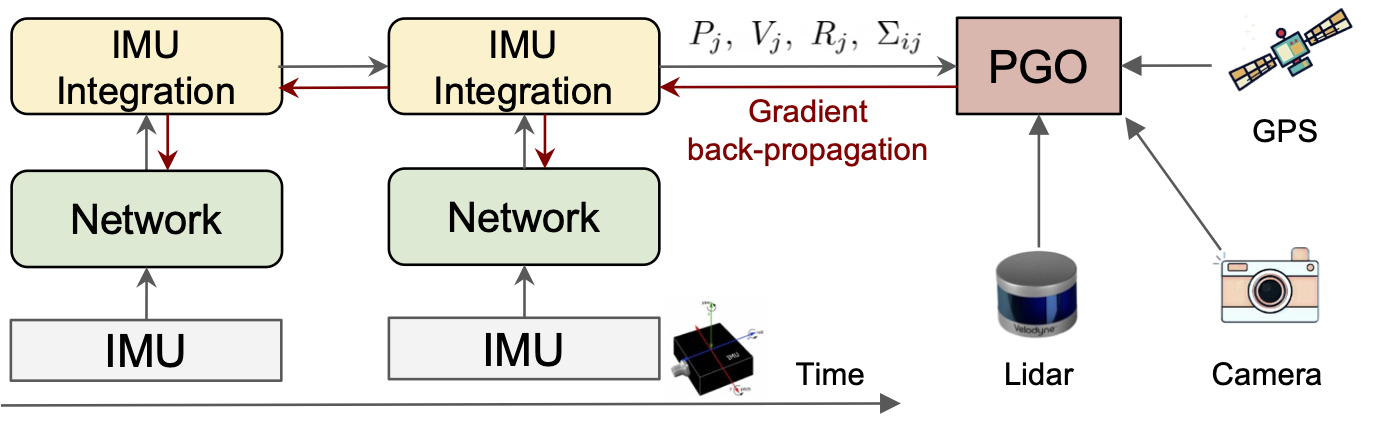
For more usage, see Documentation. For more applications, see Examples.