Motion prediction is critical for autonomous off-road driving, however, it presents significantly more challenges than on-road driving because of the complex interaction between the vehicle and the terrain. Traditional physics-based approaches encounter difficulties in accurately modeling dynamic systems and external disturbance. In contrast, data-driven neural networks require extensive datasets and struggle with explicitly capturing the fundamental physical laws, which can easily lead to poor generalization.
By merging the advantages of both methods, neuro-symbolic approaches present a promising direction. These methods embed physical laws into neural models, potentially significantly improving generalization capabilities. However, no prior works were evaluated in real-world settings for off-road driving. To bridge this gap, we present PhysORD, a neural-symbolic approach integrating the conservation law, i.e., the Euler-Lagrange equation, into data-driven neural models for motion prediction in off-road driving. Our experiments showed that PhysORD can accurately predict vehicle motion and tolerate external disturbance by modeling uncertainties. It outperforms existing methods both in accuracy and efficiency and demonstrates data-efficient learning and generalization ability in long-term prediction.
Physics-infused Model
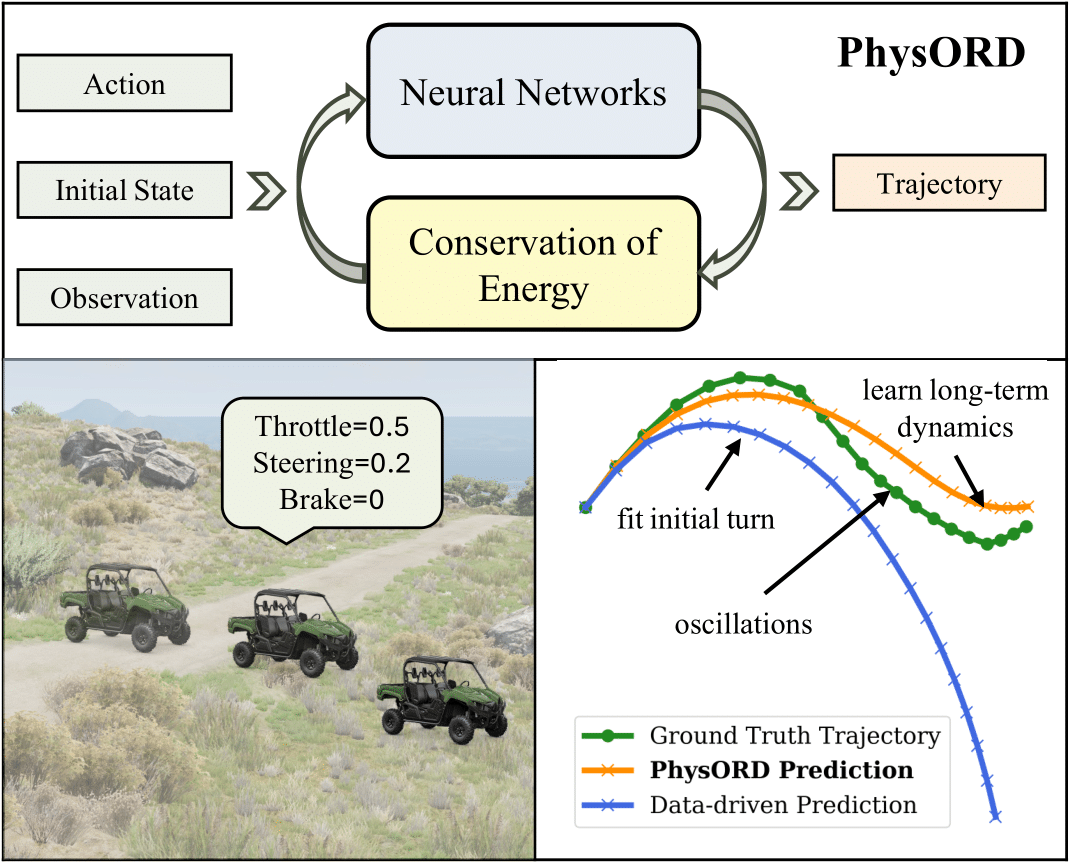
To accurately perform physics-infused forward prediction, PhysORD separates the known physical processes from less observable dynamics. This allows us to utilize symbolic reasoning to model the known physical evolution process and use data-driven components to address the unknown uncertainty.
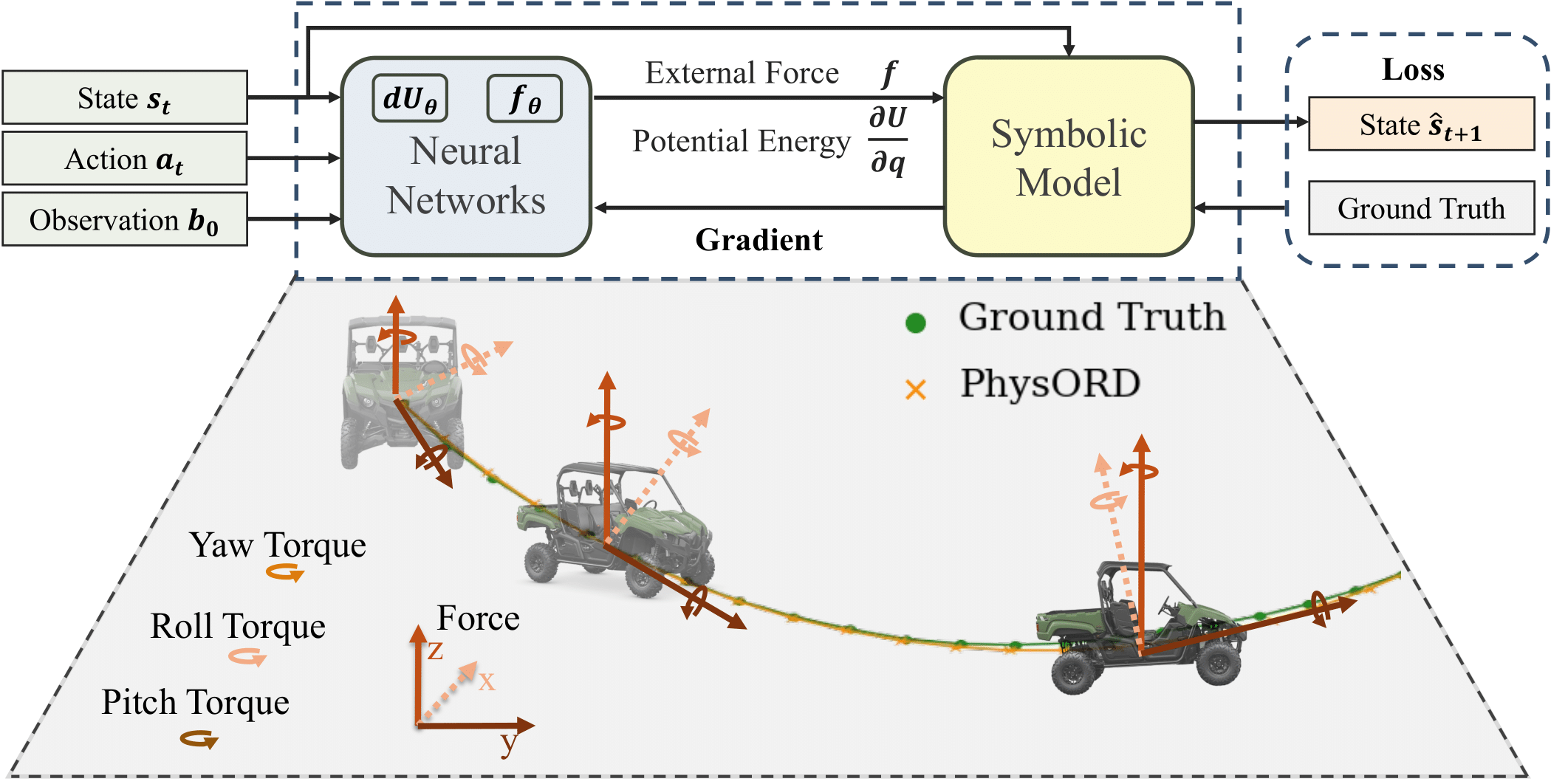
The dynamic system conserves energy and is described with the discrete Euler-Lagrange equation. However, the potential energy and external force are challenging to quantify with precision. To bridge this gap, we utilize two neural networks (\(dU_\theta\) and \(f_\theta\)) to predict these factors. The inferred values are subsequently integrated into the symbolic model, which computes the next state based on physical symbols. During the backpropagation, the symbolic model directs the gradient to refine the neural networks.
Experiments
The left figure illustrates the lowest RMSE error achieved by both TartanDrive and PhysORD within the same training duration. The right figure compares the RMSE errors for TartanDrive and PhysORD when trained with various amounts of data from 1% to 100% of training set.
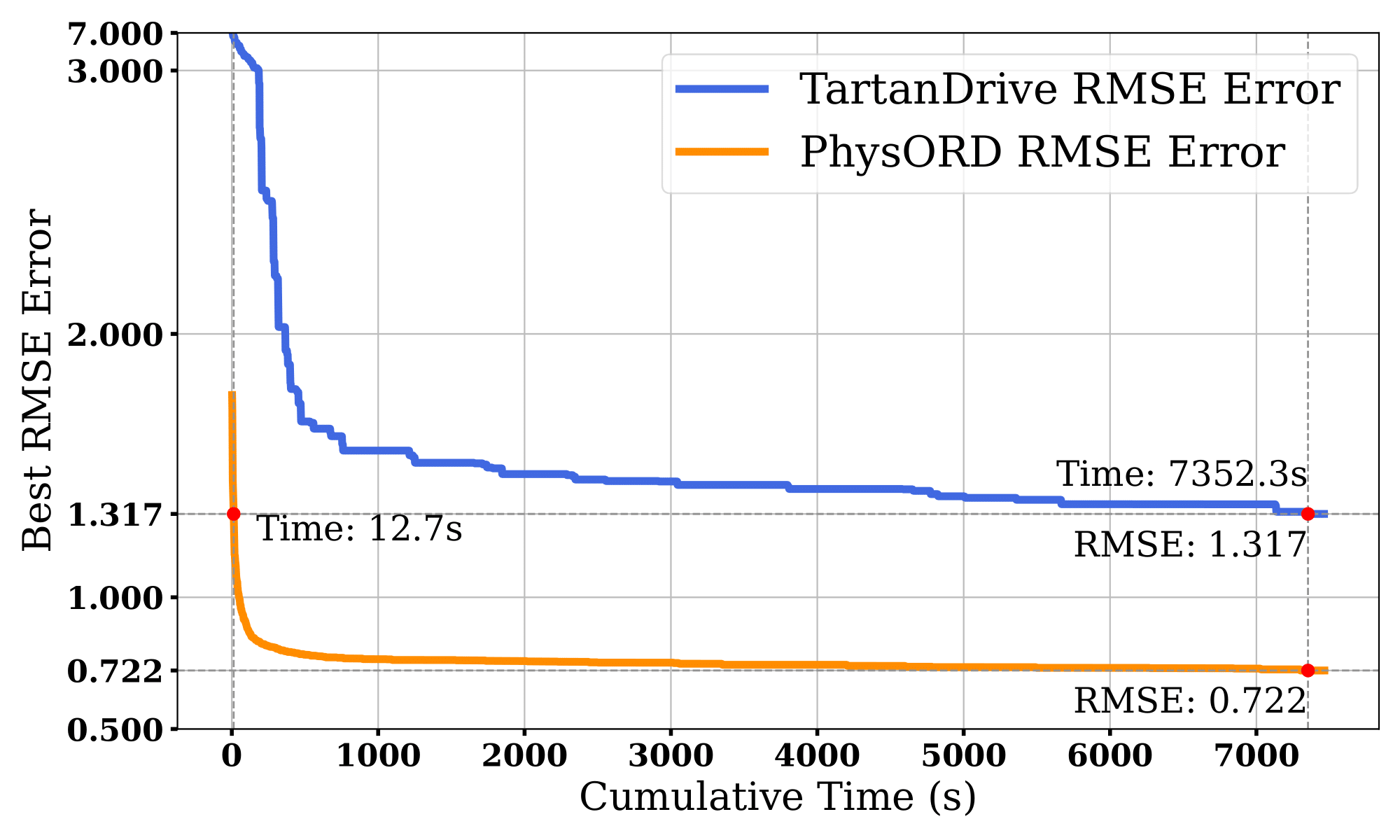
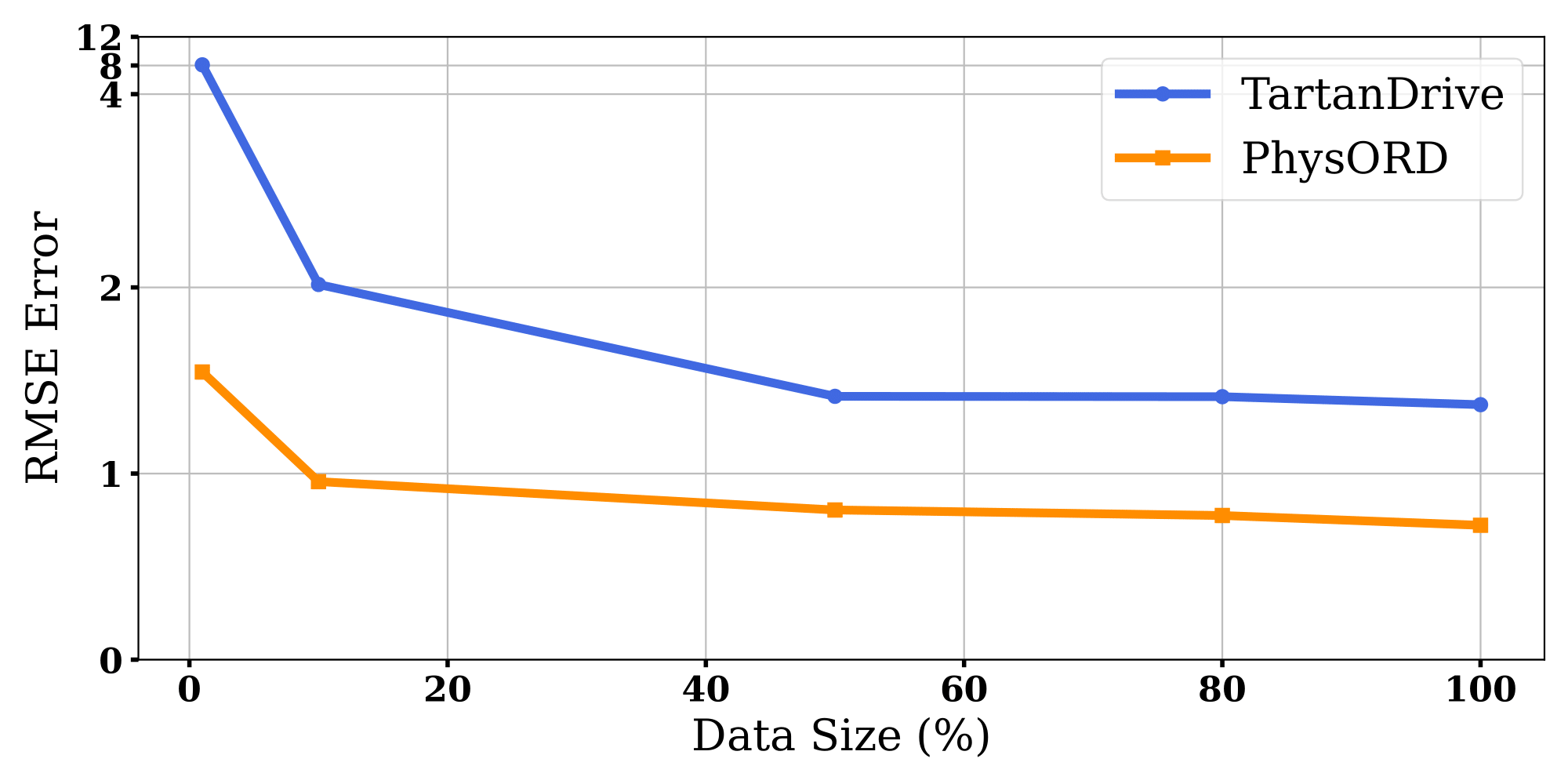
The figure below showcases qualitative comparisons of 20-step predicted trajectories covering a diverse range of distinct movements in the off-road scenario.
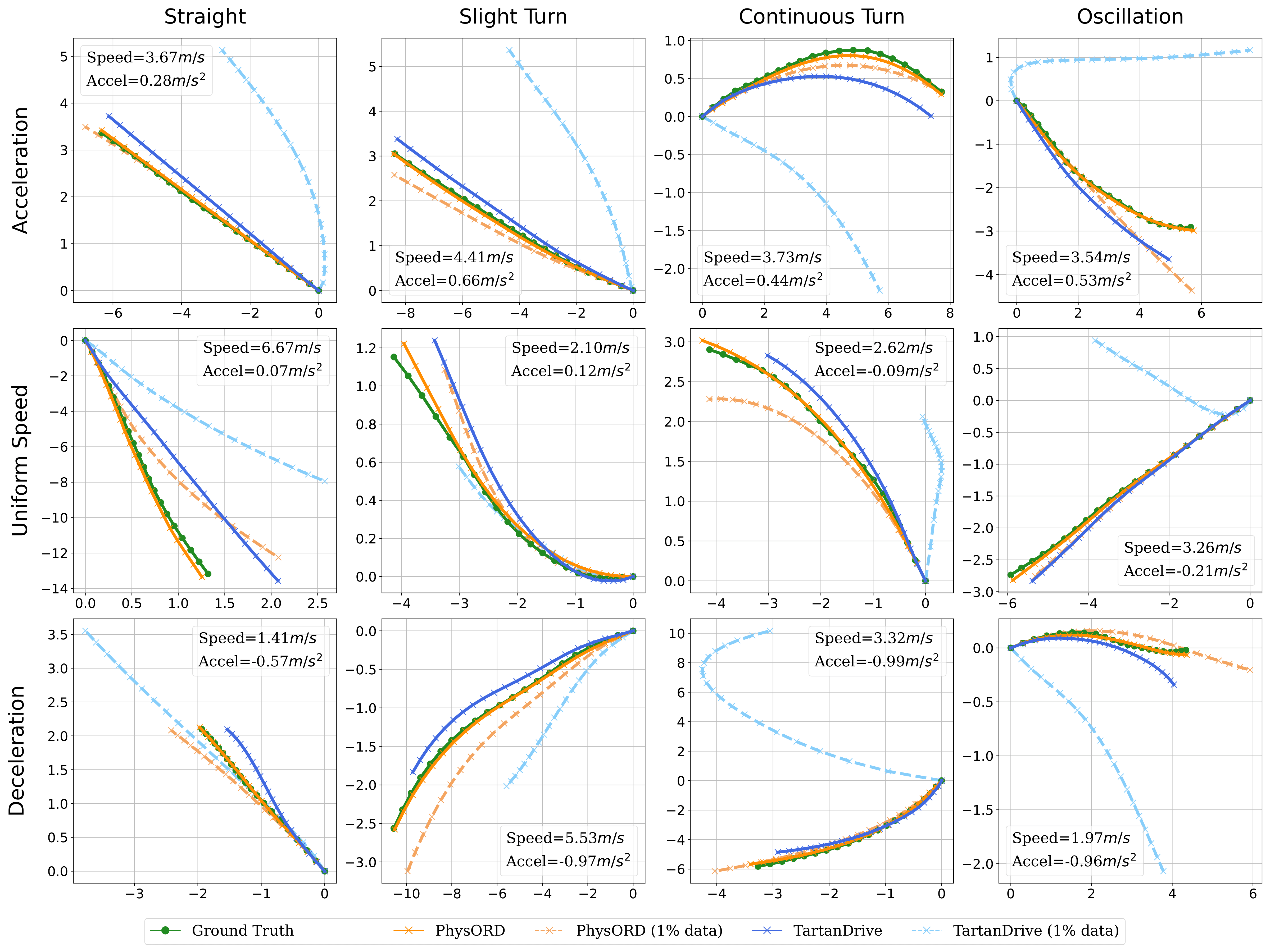
Publications
-
PhysORD: A Neuro-Symbolic Approach for Physics-infused Motion Prediction in Off-road Driving.IEEE/RSJ International Conference on Intelligent Robots and Systems (IROS), 2024.