Detecting unseen instances based on multi-view templates is a challenging problem due to its open-world nature. Traditional methodologies, which primarily rely on 2D representations and matching techniques, are often inadequate in handling pose variations and occlusions. To solve this, we introduce VoxDet, a pioneer 3D geometry-aware framework that fully utilizes the strong 3D voxel representation and reliable voxel matching mechanism.
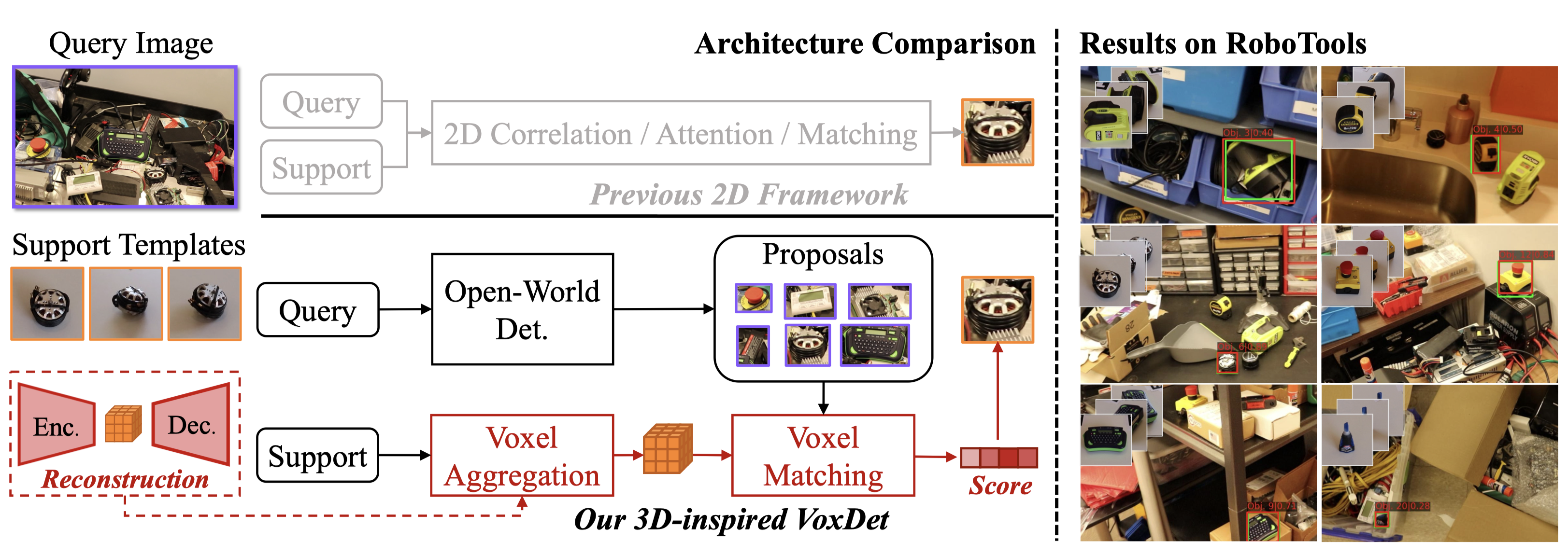
Detecting unseen instances based on multi-view templates is a challenging problem due to its open-world nature. Traditional methodologies, which primarily rely on 2D representations and matching techniques, are often inadequate in handling pose variations and occlusions.
To solve this, we introduce VoxDet, a pioneer 3D geometry-aware framework that fully utilizes the strong 3D voxel representation and reliable voxel matching mechanism. VoxDet first ingeniously proposes template voxel aggregation (TVA) module, effectively transforming multi-view 2D images into 3D voxel features. By leveraging associated camera poses, these features are aggregated into a compact 3D template voxel. In novel instance detection, this voxel representation demonstrates heightened resilience to occlusion and pose variations. We also discover that a 3D reconstruction objective helps to pre-train the 2D-3D mapping in TVA.
Second, to quickly align with the template voxel, VoxDet incorporates a Query Voxel Matching (QVM) module. The 2D queries are first converted into their voxel representation with the learned 2D-3D mapping. We find that since the 3D voxel representations encode the geometry, we can first estimate the relative rotation and then compare the aligned voxels, leading to improved accuracy and efficiency.
In addition to method, we also introduce first instance detection benchmark, RoboTools, where 20 unique instances are video-recorded with camera extrinsic. RoboTools also provides 24 challenging cluttered scenarios with more than 9k box annotations.
Contribution
- We propose the first 3D-geometry aware instance detector, VoxDet, which identifies any (novel) specifc instance in the wild.
- We develop Template Voxel Aggregation and Query Voxel Matching mechanisms to represent and match instances, respectively.
- We discover that via reconstruction pre-training, the Voxel representation is much stronger and more generalizable.
- We compile the first novel instance detection benchmark RoboTools for evaluation and synthetic training set OWID, which are all publicly awailable.
- We conduct exhaustive experiments to validate the generalization capability and robustness of VoxDet against vairous traditional 2D models.
Model Structure
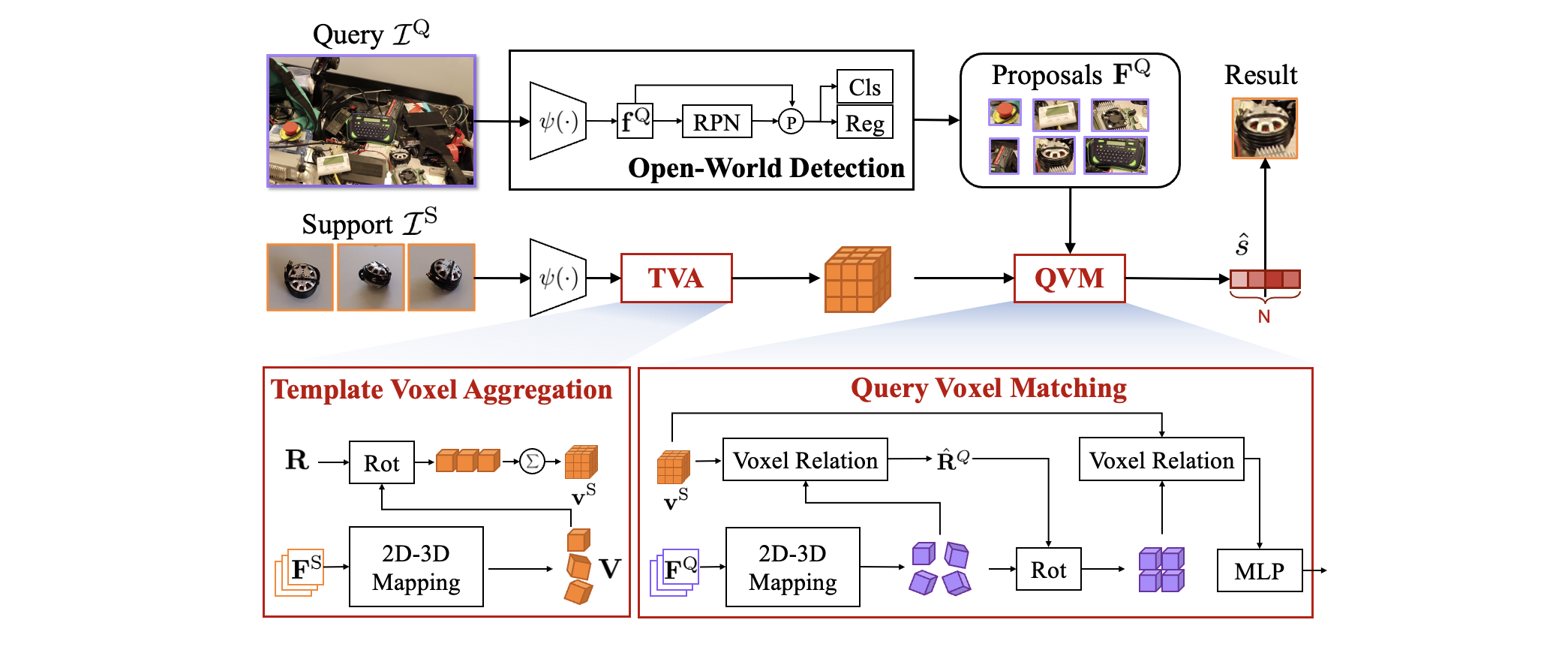
VoxDet consists of 3 main modules:
- Open-World Detection Module: Takes in an arbitrary image and outputs open-world proposals that cover all potential objects. We obtain the 2D proposal feature (ROI) to be matched.
- Template Voxel Aggregation Module: Consumes the multi-view reference images (and cooresponding camera extrinsic) as input, then construct a compact template voxel via the geometric relationship between each frame.
- Query Voxel Matching Module: Matches the template voxel with each proposal by 2D-3D mapping and voxel repation. We find such matching is tailored for instances and robust to pose variations.
Two Stage Training
We discover that two-stage training mechanism helps VoxDet learn the geometry of an instance and generalize better:
- Stage 1 Reconstruction: The 2D-3D mapping needs to construct 3D voxel from 2D image features, we discover that this module needs to be pre-trained via reconstruction objective.
- Stage 2 Detection: We first initialize the 2D-3D mapping blocks in TVA and QVM, then we used a smaller learning rate to learn the voxel representation tailored for detection task.
- (Optional) Stage 3 Rotation estimation: The rotation measurement in QVM can have additional supervision, which slightly improves performance and is optional.
Exhaustive experiments are conducted on the demanding LineMod-Occlusion, YCB-video, and RoboTools benchmarks, where VoxDet outperforms various 2D baselines remarkably with faster speed. To the best of our knowledge, VoxDet is the first to incorporate implicit 3D knowledge for 2D novel instance detection tasks.
Comparison with Gen6D
Gen6D falls short when the instance is in unseen poses or occluded.
Comparison with DTOID
DTOID can’t handel complex backgrounds very well.
Comparison with OLN_DINO
OLN_DINO has trouble distinguishing instances that have similar semantics.
Publication
-
VoxDet: Voxel Learning for Novel Instance Detection.Advances in Neural Information Processing Systems (NeurIPS), 2023.