Depth completion is crucial for many robotic tasks such as autonomous driving, 3-D reconstruction, and manipulation. However, existing methods often fail with transparent objects and real-time needs of low-power robots due to their design for opaque objects and computational intensity. To solve these problems, we propose a Fast Depth Completion framework for Transparent objects (FDCT), which also benefits downstream tasks like object pose estimation.
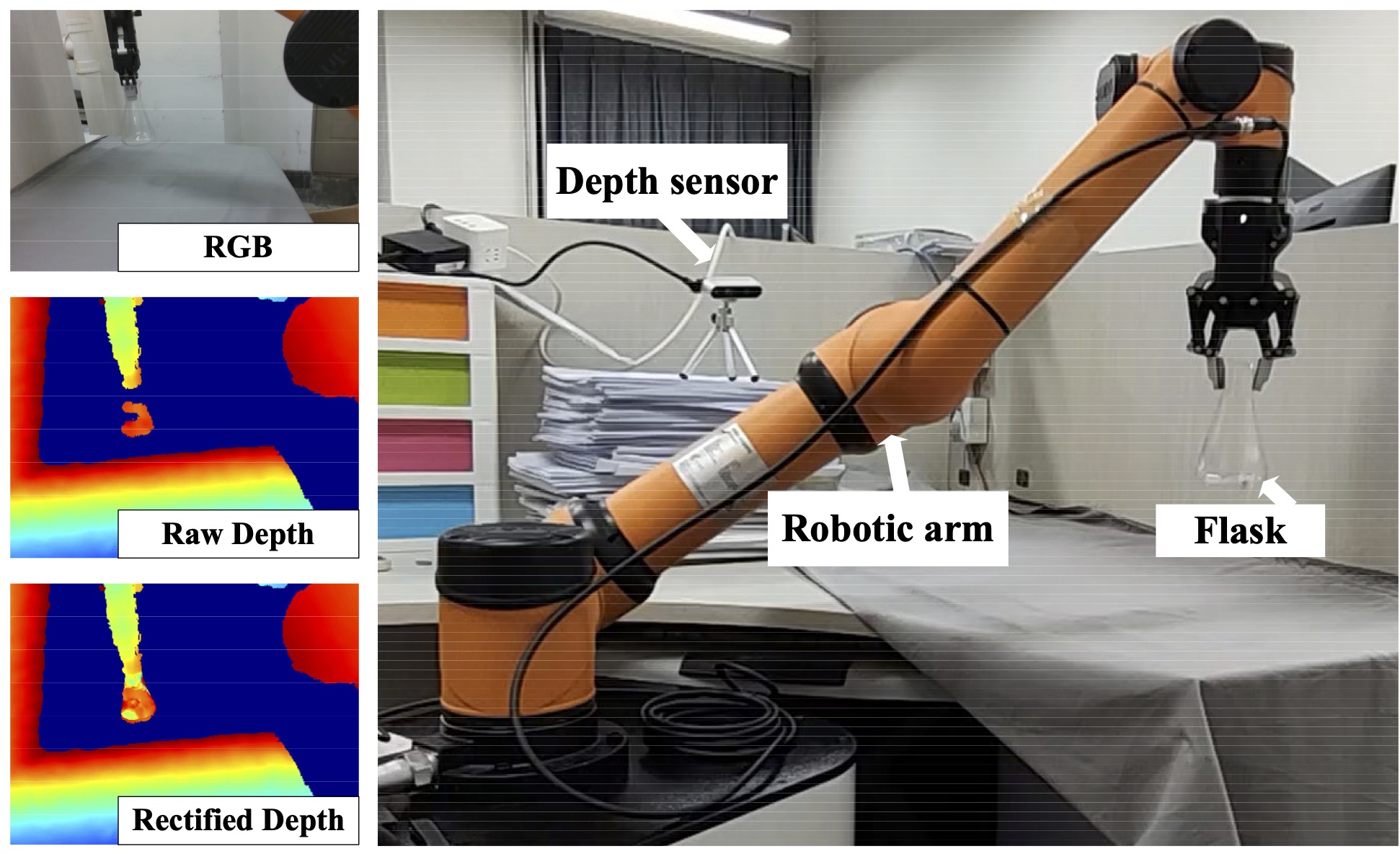
To leverage local information and avoid overfitting issues when integrating it with global information, we design a new fusion branch and shortcuts to exploit low-level features and a loss function to suppress overfitting.
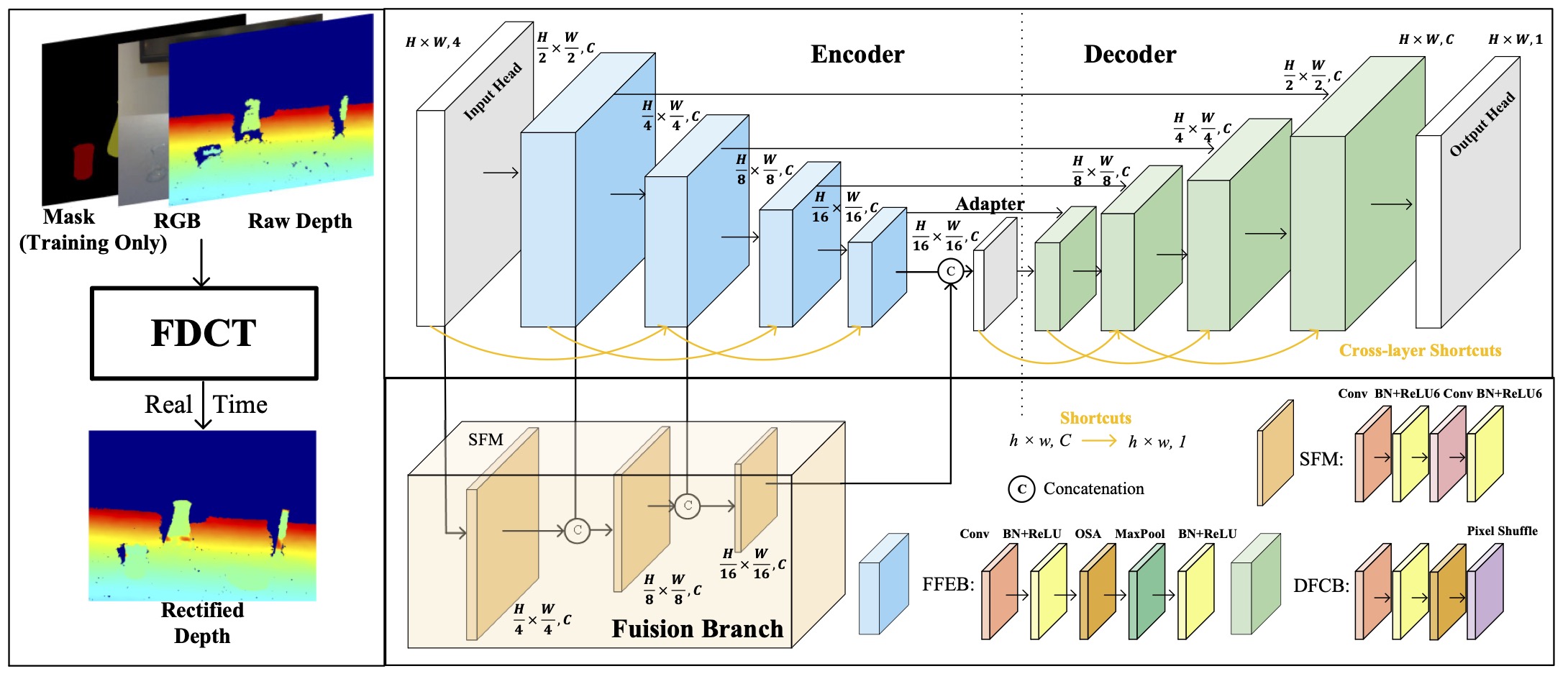
This results in an accurate and user-friendly depth rectification framework which can recover dense depth estimation from RGB-D images alone. Extensive experiments demonstrate that FDCT can run about 70 FPS with a higher accuracy than the state-of-the-art methods.
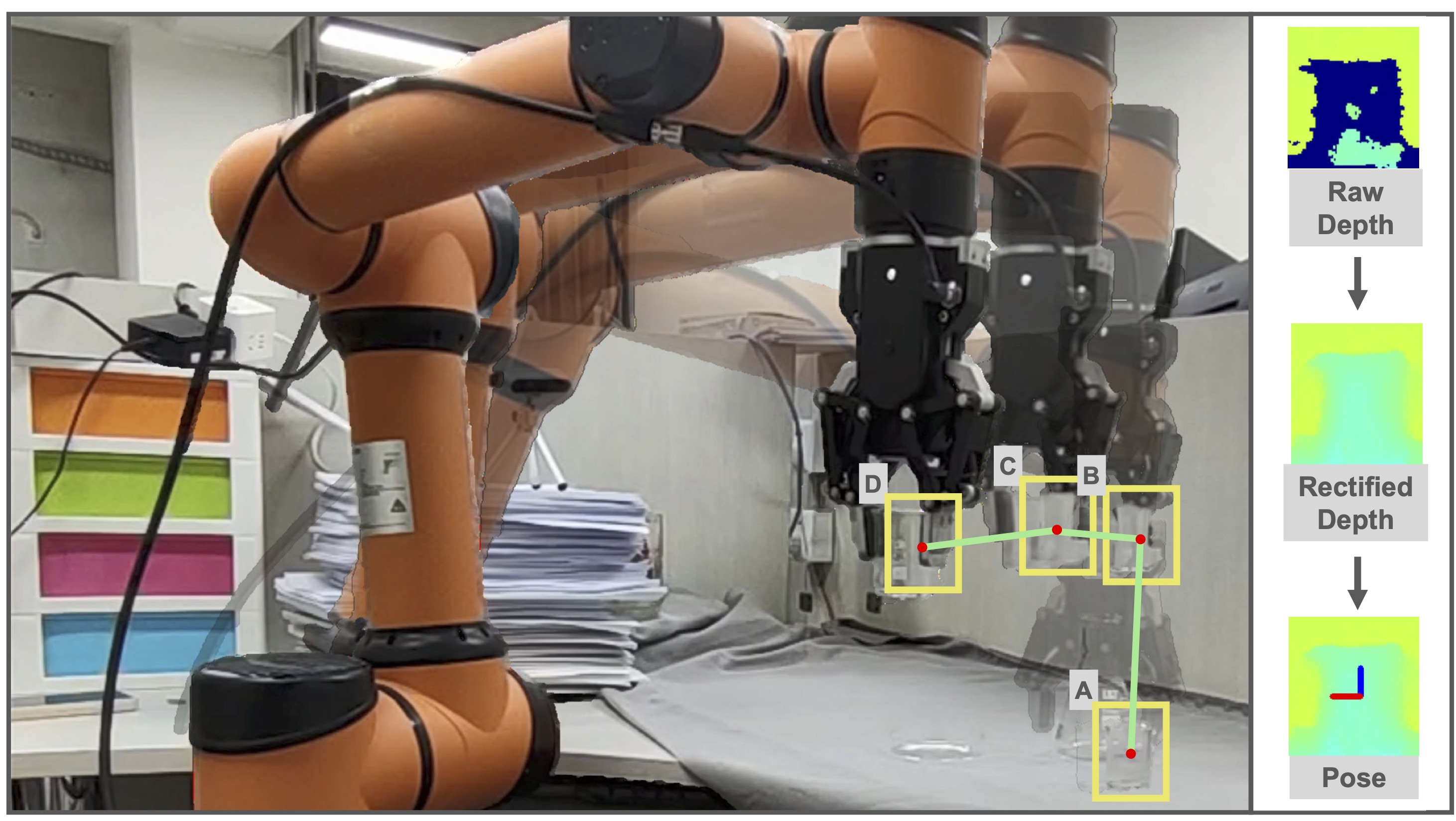
We also demonstrate that FDCT can improve pose estimation in object grasping tasks.
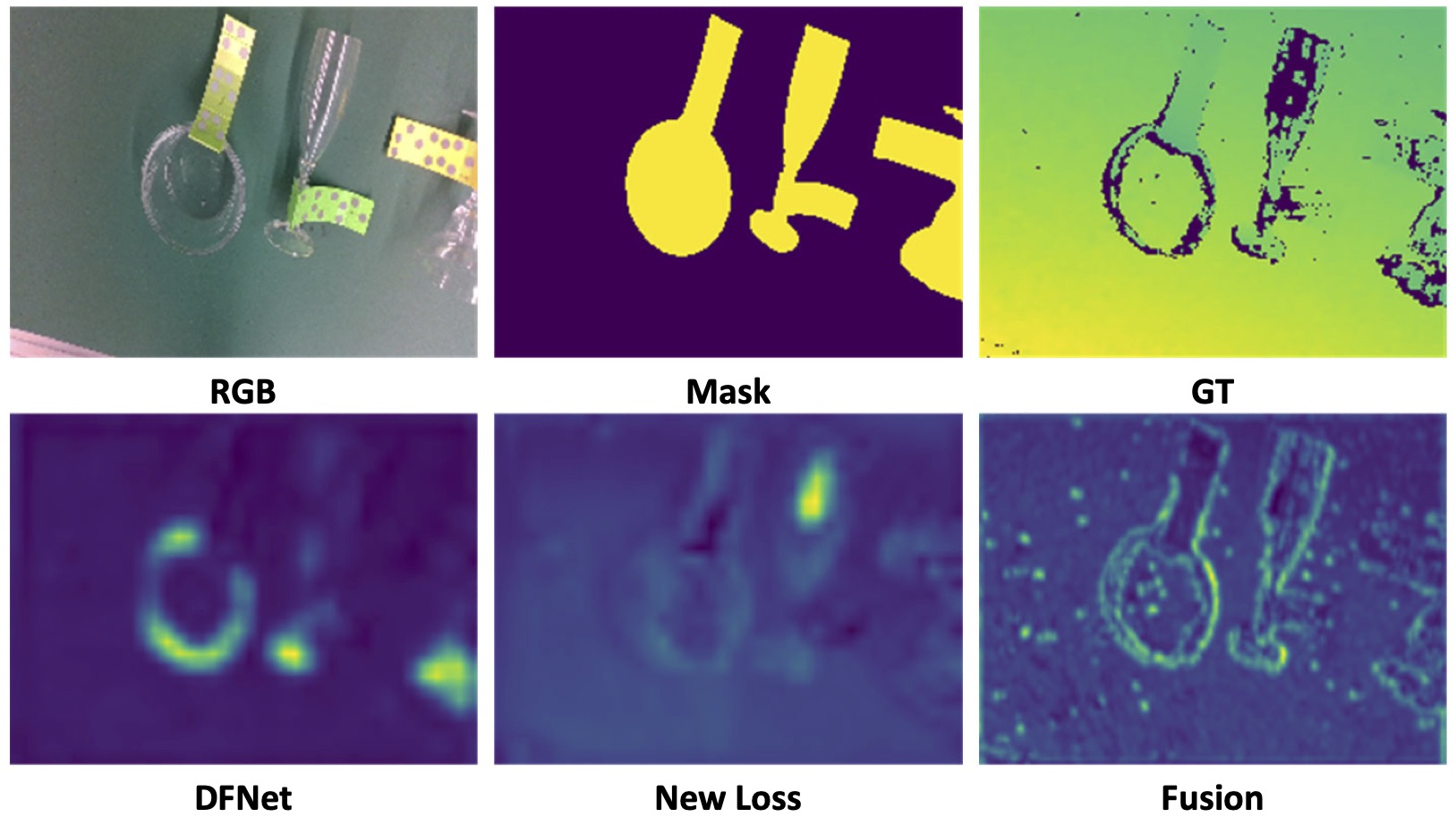
Real-time demo on transparent object manipulation.
Video
Publications
-
FDCT: A Fast Depth Completion Network for Transparent Objects.IEEE Robotics and Automation Letters (RA-L), 2023.