Indoor relocalization is vital for both robotic tasks like autonomous exploration and civil applications such as navigation with a cell phone in a shopping mall. Some previous approaches adopt geometrical information such as key-point features or local textures to carry out indoor relocalization, but they either easily fail in an environment with visually similar scenes or require many database images. Inspired by the fact that humans often remember places by recognizing unique landmarks, we resort to objects, which are more informative than geometry elements. In this work, we propose a simple yet effective object-based indoor relocalization approach, dubbed AirLoc. To overcome the critical challenges of object reidentification and remembering object relationships, we extract object-wise appearance embedding and inter-object geometric relationships. The geometry and appearance features are integrated to generate cumulative scene features. This results in a robust, accurate, and portable indoor relocalization system, which outperforms the state-of-the-art methods in room-level relocalization by 9.5% of PR-AUC and 7% of accuracy. In addition to exhaustive evaluation, we also carry out real-world tests, where AirLoc shows robustness in challenges like severe occlusion, perceptual aliasing, viewpoint shift, and deformation.
Method Overview
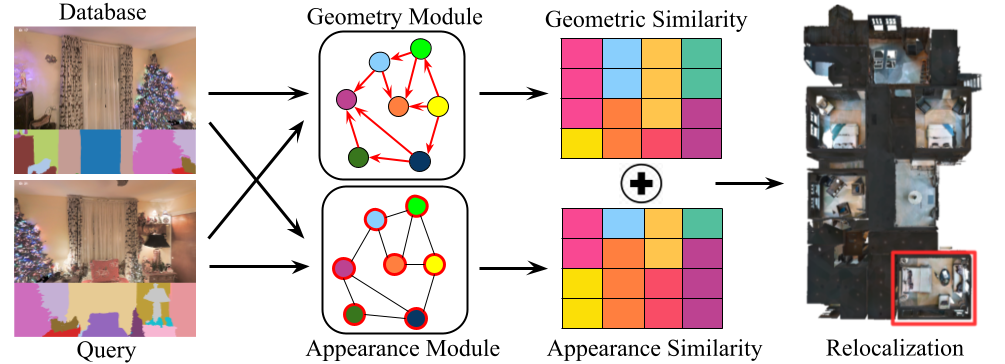
AirLoc consists of two modules: the appearance module and the geometry module. The appearance module encodes objects’ visual characteristics by extracting feature points from the objects and aggregating them to form a collective object encoding using a modified version of the NetVLAD framework. The similarity between query and database objects is then calculated using the cosine similarity. The geometry module, on the other hand, uses the relative positions of the objects to assist in the appearance-based matching. It does this by encoding geometric information using the mean location of object-wise key-points. These two modules are then ensembled to improve the relocalization accuracy.
Experimental Results
Quantitative Results:
Precision-Recall plots showing comparison between AirLoc and different baselines for different K values. Here K is the number of images taken per room as a database.
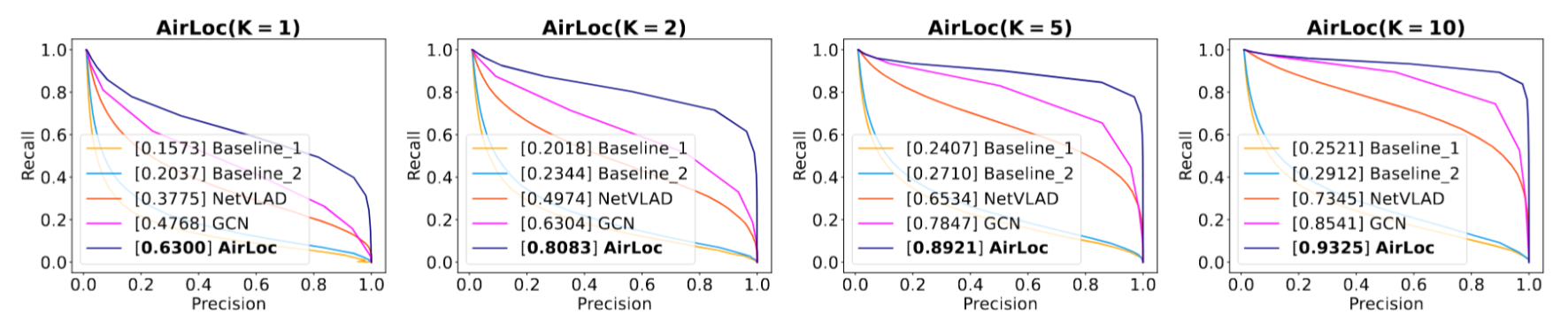
Qualitative results:
For a query, the closest database image produced by NetVLAD is shown in the right column, while the closest database image produced by AirLoc is shown in the middle column.
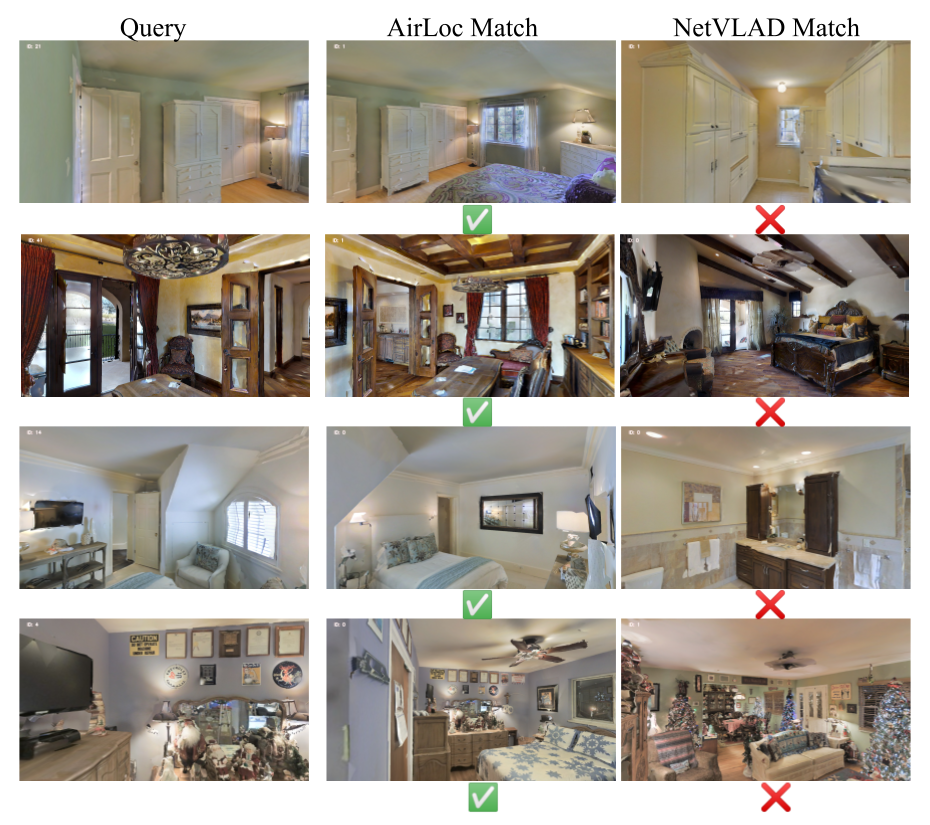
Real-World Demo:
The left side displays the corresponding query captured by a mobile phone, while the right side shows the relocalization result.
Publications
-
AirLoc: Object-based Indoor Relocalization.arXiv preprint arXiv:2304.00954, 2024.